목록분류 전체보기 (307)
On the journey of
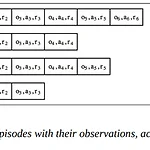
Deep Reinforcement Learning 내용정리는 파알 신입기수 때(...무려 1년 전) 공부하면서 노션에 정리한 내용을 복습하며 티스토리로 옮겨온 내용입니다. 때문에 학회 노션에 최적화된 구조와 내용임을 밝혀둡니다 모종의 이유로 학회활동 당시 Chapter 3는 공부하지 않았었는데 왜 그랬는지는 잘 모르겠습니다 The Cross-Entropy Method DQN or Advantage Actor-Critic과 같은 다른 도구보다 훨씬 덜 유명하지만, 자체적인 강점이 있다 단순성: 교차 엔트로피 방법은 매우 간단 → 직관적인 방법 양호한 수렴 복잡한 다단계 정책을 학습하고 발견할 필요가 없음 rewards가 빈번한 짧은 에피소드를 가진 단순한 환경에서는 교차 엔트로피가 일반적으로 매우 잘 작동..
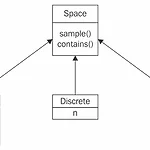
2.1 The Anatomy of the Agent 2.1.1 A Simplistic Situation Define an environment that gives the agent random rewards for a limited number of steps, regardless of the agent’s actions class Environment: def __init__(self): self.steps_left = 10 # initialize its internal state def get_observation(self): return [0.0, 0.0, 0.0] def get_actions(self): return [0, 1] # agent가 실행할 수 있는 동작의 집합 def is_done(s..
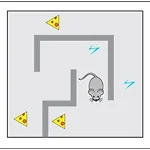
Chapter 1. What is Reinforcement Learning? 💡 Reinforcement Learning (RL) : an approach that natively incorporates extra dimension (which is usually time, but not necessarily) into learning equations 강화학습 : 어떤 환경 안에서 정의된 에이전트가 현재의 상태를 인식하여, 선택 가능한 행동들 중 보상을 최대화하는 행동 혹은 행동 순서를 선택하는 방법을 통해 학습 Learning - supervised, unsupervised, and reinforcement 지도, 비지도 학습과 비교를 통해 강화 학습의 특징을 정의 supervised learning..
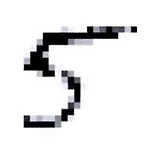
3.1 MNIST 💡 MNIST 데이터셋 (Modified National Institue of Standards and Technology Dataset) : 고등학생과 미국 인구조사국 직원들이 손으로 쓴 70,000개의 작은 숫자이미지를 모은 데이터셋 머신러닝 분야의 ‘Hello World’와 같은 학습용 데이터셋 MNIST 데이터셋 가져오기 ‘mnist_784’ : id version=1 : 하나의 id에 여러 개의 버전이 있음 출력값 {'COL_NAMES':['label', 'data'], 'DESCR': ... 'data' : array([[0, 0, 0, ..., 0, 0, 0], [0, 0, 0, ..., 0, 0, 0], [0, 0, 0, ..., 0, 0, 0], ... [0, 0, 0,..