목록Experiences & Study/VQA (8)
On the journey of
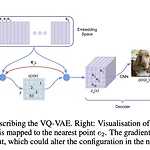
Original paper ) https://arxiv.org/abs/1711.00937 Neural Discrete Representation Learning Learning useful representations without supervision remains a key challenge in machine learning. In this paper, we propose a simple yet powerful generative model that learns such discrete representations. Our model, the Vector Quantised-Variational AutoEnc arxiv.org https://arxiv.org/pdf/1711.00937.pdf (Dir..
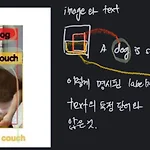
Original Paper ) https://arxiv.org/pdf/2004.06165.pdf Introduction & Background : 이전 VLP에 대해 VLP는 self-supervised learning으로 cross-modal representation을 학습한다 기존의 Transformer 기반의 연구들은 제한들이 vision 영역에서 해결되지 못한 부분들이 있다. 모호성(ambiguity) : image 내에서 2개의 class/object가 겹쳐있는 경우가 많다. 이 때에 대한 해결성이 조금은 애매하다는 문제가 있다. Lack of grounding : image내의 object와 text사이에서 정확하게 명시된 labeling된 어떠한 값이 존재하지 않는다는 의미. 이것은 wea..
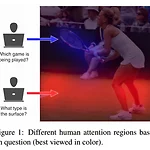
Original Paper) https://arxiv.org/abs/1606.03556 Human Attention in Visual Question Answering: Do Humans and Deep Networks Look at the Same Regions? We conduct large-scale studies on `human attention' in Visual Question Answering (VQA) to understand where humans choose to look to answer questions about images. We design and test multiple game-inspired novel attention-annotation interfaces that r..
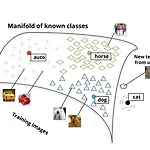
Original Paper ) https://arxiv.org/abs/1301.3666v2 Zero-Shot Learning Through Cross-Modal Transfer This work introduces a model that can recognize objects in images even if no training data is available for the objects. The only necessary knowledge about the unseen categories comes from unsupervised large text corpora. In our zero-shot framework distrib arxiv.org 전에 MeLU 추천시스템에 대해 포스팅한 적이 있었다(같은..