목록Vision (3)
On the journey of
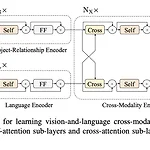
Original Paper ) https://arxiv.org/abs/1908.07490 LXMERT: Learning Cross-Modality Encoder Representations from Transformers Vision-and-language reasoning requires an understanding of visual concepts, language semantics, and, most importantly, the alignment and relationships between these two modalities. We thus propose the LXMERT (Learning Cross-Modality Encoder Representations arxiv.org 깔끔하게 읽혀..
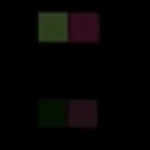
* 해당 포스팅은 Attention 구조 및 Transformer에 대한 논의를.. 좀더 잘 이해하기 위해 공부하고 쓰는 글입니다. URP에서 본격적으로 다룬 내용은 아님을 밝혀둡니다 :) References(Github & Huggingface) https://nlpinkorean.github.io/illustrated-transformer/ https://github.com/hyunwoongko/transformer/blob/master/models/layers/multi_head_attention.py https://github.com/rwightman/pytorch-image-models/blob/a520da9b495422bc773fb5dfe10819acb8bd7c5c/timm/models/vis..
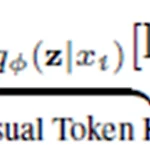
2.4 From the Perspective of Variational Autoencoder BEIT 의 pre-training은 Variational Autoencoder 관점에서 설명할 수 있음 이를 분포를 기반으로 한 수식으로 나타내보면 아래와 같다. 이때 위 수식은 아래와 같이 변형될 수 있다. Stage 1 : dVAE에서 Image Tokenizer를 얻는 부분에 대한 Term Stage 2 : Masked Image가 주어졌을 때, Image Tokenizer를 얻는 것에 대한 Term 2.5 Pre-Training Setup BEIT 모델의 Pre-Training은 아래와 같이 설정되고 진행됨 VIT-B(Base) 모델 설정과 비슷하게 설정되어 있음 12-layer Transformer w..