목록VQA (7)
On the journey of
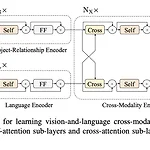
Original Paper ) https://arxiv.org/abs/1908.07490 LXMERT: Learning Cross-Modality Encoder Representations from Transformers Vision-and-language reasoning requires an understanding of visual concepts, language semantics, and, most importantly, the alignment and relationships between these two modalities. We thus propose the LXMERT (Learning Cross-Modality Encoder Representations arxiv.org 깔끔하게 읽혀..
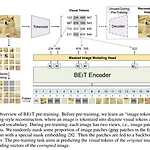
Original Paper ) BEiT: https://arxiv.org/abs/2106.08254 BEiT: BERT Pre-Training of Image Transformers We introduce a self-supervised vision representation model BEiT, which stands for Bidirectional Encoder representation from Image Transformers. Following BERT developed in the natural language processing area, we propose a masked image modeling task to pre arxiv.org Contribution BERT의 Masked Lan..
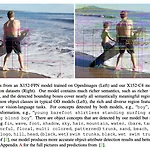
Original Paper) https://openaccess.thecvf.com/content/CVPR2021/html/Zhang_VinVL_Revisiting_Visual_Representations_in_Vision-Language_Models_CVPR_2021_paper.html CVPR 2021 Open Access Repository VinVL: Revisiting Visual Representations in Vision-Language Models Pengchuan Zhang, Xiujun Li, Xiaowei Hu, Jianwei Yang, Lei Zhang, Lijuan Wang, Yejin Choi, Jianfeng Gao; Proceedings of the IEEE/CVF Confe..
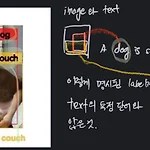
Original Paper ) https://arxiv.org/pdf/2004.06165.pdf Introduction & Background : 이전 VLP에 대해 VLP는 self-supervised learning으로 cross-modal representation을 학습한다 기존의 Transformer 기반의 연구들은 제한들이 vision 영역에서 해결되지 못한 부분들이 있다. 모호성(ambiguity) : image 내에서 2개의 class/object가 겹쳐있는 경우가 많다. 이 때에 대한 해결성이 조금은 애매하다는 문제가 있다. Lack of grounding : image내의 object와 text사이에서 정확하게 명시된 labeling된 어떠한 값이 존재하지 않는다는 의미. 이것은 wea..